Accurate identity judgements are critical in ensuring that suspects can be apprehended by law enforcement and national security agencies, and that identity fraud attacks do not go undetected at border control points. Research has shown that typical human observers are poor at facial recognition in these contexts. However, there is now a decade’s worth of psychological science which shows that some individuals – known as super-recognizers – excel at such tasks. This article reviews the latest super-recognition science for agencies to consider implementing to enable a powerful and cost-effective identity verification advantage.
Introduction
Police have long been aware of the fallibility of eyewitness memory and subsequent testimony. In over 70 percent of 365 DNA-exoneration cases, innocent defendants were identified by mistaken witnesses [1]. More routinely, 25 percent of witnesses identify known-innocent foils from United States (U.S.) and United Kingdom (U.K.) line-ups, despite instructions that the perpetrator may not be present [2]. Closed circuit television (CCTV) implementation was marketed as a solution, allowing permanent crime scene image retention to facilitate suspect identification without necessarily having to draw on human memory. Even with low-quality images, highly familiar face recognition is normally reliable [3], although suspect familiarity will vary (i.e. since last encounter). However, most police officers are unfamiliar with most suspects, and unfamiliar face recognition is highly prone to error, even when high-quality images are available [4]. Recent research, however, has demonstrated large individual differences in unfamiliar face recognition ability [5], with those at the top end labelled as ‘super-recognizers’ (SRs) [6]. Over the past 10 years, a small number of international police forces, identity verification organizations (i.e. border control), and businesses have deployed SRs to take advantage of their superior facial identity verification skills [7, 8].
Establishing The Super-recognizer Advantage
While the first scientific study on super-recognition was published in 2009 [6], it was not until April 2011 that real-world cases of super-recognition within a policing context were first detected. The lead author, working in collaboration with London’s Metropolitan Police Service (‘the MET’), found that a particular set of officers were making frequent and highly accurate suspect identifications (‘idents’) from CCTV images captured across London. Subsequent research on these SRs by Davis et al. [7] and Robertson et al. [8] found that they outperformed typical face recognisers on a number of facial recognition tests. These tests used both familiar, learned, and unfamiliar faces, and which tapped memory for faces (i.e. recognizing a suspect from CCTV) and simultaneous face matching (i.e. deciding whether the face of the individual in the interview room matched the face of the suspect held on file). Since 2016, a number of additional peer-reviewed scientific studies have shown that the SR advantage is sustained even if the ethnicity of the target identity is not that of the SR observer [9] (see Fig. 1), if the targets are very young children [10], or are placed within complex visual scenes such as crowd videos [11]. Superior performance in SRs appears to be a face-specific and due to heritable individual differences unrelated to experience or training (i.e. we cannot train typical recognisers to be SRs [12, 13]).
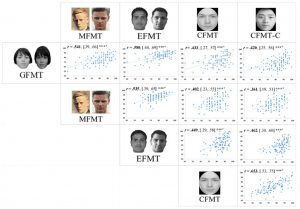
Figure 1: Data from [9] showing that individuals at the top end of the facial recognition ability spectrum excel across a variety of face-based tasks. This ability remains even when the target face is from a different ethnic group than that of the observer (Glasgow Face Matching Test (GFMT), Models Face Matching Test (MFMT), Egyptian Face Matching Test (EFMT) – unfamiliar face matching; Cambridge Face Memory Test (CFMT), Cambridge Face Memory Test-Chinese version (CFMT-C) – learned face memory).
Super-recognizers’ Successes in Policing
Following the research described above [7], 20 of the MET SRs recruited to the study made more than 600 idents of often-disguised London rioters after lawlessness erupted across the city in August 2011. One SR correctly identified 180 suspects [14]. These MET SRs had rarely met rioters in person, or if familiar, had sometimes not encountered them for many years. In these cases, rioters had been tracked through different CCTV feeds to extract the best quality image for matching against mugshot databases. Most SR-identified rioters were convicted (> 70 percent), after inculpating evidence was secured, such as stolen property, confessions, or clothing matching that seen in the CCTV images. This success, which was generated simply by identifying existing officers within the force who excelled at facial recognition, was followed up by expanded testing and the identification of more MET SRs. A full-time New Scotland Yard Super-Recognition Unit became operational in May 2015. A German SR unit has now also been set up in Munich by the Bavarian State Police after similar testing of 5000 police officers (see [15] for a review).
The MET statistics reported in the media [16] show that this new Super-Recognition Unit led to substantially increased identification rates, as well as prosecutions and convictions for volume (theft, robbery) and highly serious crimes (murder, attempted murder, rape). MET SR Unit officers accomplished this by matching new images with those stored in a central repository of London’s unsolved crimes. Other MET SRs worked in front line roles. Prioritized viewing of images of crime-types for which they were an expert or those from their vicinity resulted in multiple familiar suspect identifications. MET SRs sometimes committed to memory large numbers of facial photos of suspects prior to large public events, aiming to recognize them in the crowds. Others reported spontaneously spotting wanted fugitives, for instance, on public transport while off-duty. While the SRs do have an exceptional talent for facial identification, they, like automatic facial algorithms [17] are not infallible. It is not possible to estimate how many suspects they missed in similar circumstances. Nevertheless, using SRs, identified through short scientific tests which can be completed online to ensure that frontline police time is not affected, can significantly improve suspect identification rates in a variety of contexts.
Super-recognizer’s in Border Control/Facial Image Matching Contexts?
As outlined above, the scientific basis allied with case study support from the MET suggests that introducing SR units would be advantageous to all police forces. SRs are also likely to enhance the detection of identity fraud attacks at border control points (or indeed any identity task in which one has to match a face to a face photo in an identity document). At border control, passport checking officials are required to match the face of an unfamiliar traveller standing in front of them, to the face photo in their passport. Typical recognizers perform poorly at this task with typical error rates in ideal viewing conditions of around 10 percent, which is a non-trivial level of error [18]. Identity fraudsters seeking to enter a country illegally will often present a stolen passport showing an individual to whom they bear a likeness. Checking officials must detect when the faces mismatch, and research has shown that SRs are also likely to excel at this task [7]. SRs are more likely to spot a fraud attack in which a fraudster’s face and the passport photo they present actually show two different, but similar looking, individuals.
Are There Limitations to Super-recognizer’s Skills?
There are important limitations to SR’s abilities, however. First, the SR advantage appears to be specific to faces [13]. SRs perform no better at identifying non-face objects (e.g. cars) than typical recognizers. Such individuals would be likely of little use in supporting the recovery of stolen vehicles or other goods. Second, only two percent of the population possess the SR ability as it is currently defined. However, dependent on task, workplace operations may also be enhanced by recruiting those at the ‘top end of typical’, while redeploying poor recognizers to non-identity based tasks. Third, recent research suggests that identity recognition performance is not connected to the ability to detect hyper-realistic face masks [9, 19]. Also, only small correlations have been reported between identity verification accuracy and the ability to detect fraudulent passport morphs (e.g., [20]).
What About Facial Recognition Algorithms?
In terms of morph and hyper-realistic mask fraud attacks, computerized face recognition algorithms may be more accurate (e.g. for morph detection, see [21]). Indeed, in many operations, such as passport checking at border control, in which thousands of daily identity verification decisions are required, algorithms facilitate fast accurate checking of the passports of most lawful travellers. However, current facial recognition algorithms, like SRs, will not always provide perfect levels of identity verification performance [22, 23]. More generally, concerns have been raised by privacy advocates, politicians and the public about their indiscriminate use in other types of public space [24]. National Institute of Science and Technology appraisals have shown that some systems are more likely to misidentify members of specific ethnic groups [25]. Furthermore, highly publicized police tests in the street and at sports stadiums in the United Kingdom resulted in police questioning innocent people, wrongly identified as being fugitives from justice [26]. Most errors were quickly rectified following human review – and this is the conundrum. In legal settings, it is human system operators — police officers, or jury members — who determine identity, and not the algorithms. This has led to a call to pair our current best performing algorithms with SRs to achieve current best possible performance.
How Can We Best Achieve Best Possible Identity Verification Right Now?
Algorithm performance can be predicted by certain factors. These include image quality, changes in physical appearance of targets (i.e. age, skin tone, facial hair) and most importantly the size of the Photo-ID database against which a target image is being compared, and the associated risk of doppelgänger identification [26]. Only one study has directly compared algorithms and SRs at one-to-one matching of twenty pairs of high-quality facial images previously identified as being extremely hard to match [17]. The performance of the top-performing commercial algorithm matched the mean scores of the SRs, with both significantly outperforming controls. Intriguingly, the fusion of algorithm and SR decision-making resulted in the highest levels of accuracy. This effect is similar to the wisdom of the crowd paradigm.
Amalgamating independent simultaneous face matching decisions from individuals in order to form a ‘crowd,’ is more accurate than individual decisions alone (e.g. [27]). Davis et al. [28] showed that face matching accuracy may be further enhanced when the crowd is made up of SRs. After forensic facial examiners declined to assist an investigation because the key image was not of sufficient quality, the authors assisted police in verifying identification of a 1970’s facial photograph of a drowned man. They created a line-up containing a photo of a man who was reported missing to police at about the same time (the target) and seven foils depicted in contemporary photos. These foils were of the same ‘age, appearance and position in life’. Compared to individual police controls and SRs, and to a crowd of police controls, a crowd of police SRs was more likely to confidently match the deceased and target photo. A coroner ruled that this case study, and other documents provided sufficient evidence to allow a death certificate to be issued on the assumption that both photos depicted the same person.
From Science to Society: How Can Police Forces/Security Agencies Select and Implement Super-recognizer Teams?
The logical approach, and the prevailing view within the literature, is that SRs must be selected on the basis of consistently high scores across a battery of facial recognition tests. Such tests must reflect the types of identity checks, interfaces, ages, ethnicities, time pressure, and work pattern factors that the officer or official is likely to encounter on the job (e.g. [29]). Applied cognitive science has a battery of tests that could assist police forces or government agencies in identifying potential SRs. Then, new job-specific tasks would be created which match, as closely as possible, the real-world role/interface. Only prospective SRs who perform well on the existing tests, and who have their SR status confirmed by performance on specific tasks should be recruited for that role. For organizations seeking to increase the pool of top-level identity checkers, the ‘top end of typical’ could also be recruited in the same way. It is important not to sacrifice significant improvements, through the selection of SRs and better-than average performers coupled with the redeployment of poor recognizers, in the search for perfection (i.e. SR-only units).
Conclusion
This article has provided a short review of the latest in SR science and provided some examples of real-world SR successes. There are limitations to the abilities of SRs, but there is now strong evidence which supports more widespread consideration of SRs among police forces and security agencies. Pairing SRs with our best algorithms is the most likely approach to provide superior levels of performance. Working with psychological science, police forces and security organizations will find support for the implementation of SRs, and at lower cost compared to automated systems.
References
- Innocence Project (2019). Exoneration statistics and databases. Retrieved 15 March 2020 from, https://www.innocenceproject.org/exoneration-statistics-and-databases/
- Horry, R., Memon, A., Wright, D., & Milne, R. (2012). Predictors of eyewitness identification decisions from video lineups in England: A field study. Law and Human Behavior, 36(4), 257-265.
- Burton, A. M., Wilson, S., Cowan, M., & Bruce, V. (1999). Face recognition in poor quality video: Evidence from security surveillance. Psychological Science, 10, 243–248.
- Burton, M., White, D., & McNeill A. (2010). The Glasgow face matching test. Behavior Research Methods, 42, 286-291.
- Tardiff, J., Morin Duchesne, X., Cohan, S., Royer, J., Blais, C., Fiset, D., Duchaine, B., & Gosselin, F. (2019). Use of face information varies systematically from developmental prosopagnosics to super-recognizers. Psychological Science, 30(2), 300-308.
- Russell, R., Duchaine, B., & Nakayama, K. (2009). Super-recognizers: People with extraordinary face recognition ability. Psychonomic Bulletin & Review, 16, 252-257.
- Davis, J. P., Lander, K., Evans, R., & Jansari, A. (2016). Investigating predictors of superior face recognition ability in police super-recognisers. Applied Cognitive Psychology, 30(6), 827-840.
- Robertson, D. J., Noyes, E., Dowsett, A. J., Jenkins, R., & Burton, A.M. (2016). Face recognition by Metropolitan Police super-recognisers. PLOS, One, 11(2): e0150036.
- Robertson, D. J., Black, J., Chamberlain, B., Megreya, A. M., & Davis, J. P. (2020). Super-recognisers show an advantage for other race face identification. Applied Cognitive Psychology, 34(1), 205-216.
- Belanova, E., Davis, J. P., & Thompson, T. (2018). Cognitive and neural markers of super-recognisers’ face processing superiority and enhanced cross-age effect. Cortex, 108, 92-111.
- Davis, J. P., Forrest, C., Treml, F., & Jansari, A. (2018). Identification from CCTV: Assessing police super-recogniser ability to spot faces in a crowd and susceptibility to change blindness. Applied Cognitive Psychology, 32(3), 337-353.
- Towler, A., Kemp, R. I., Burton, A. M., Dunn, J. D., Wayne, T., Moreton, R., & White, D. (2019). Do professional facial image comparison training courses work? PLOS One, 14(2), e0211037.
- Wilmer, J. B., Germine, L., Chabris, C. F., Chatterjee, G., Williams, M., Loken, E., … & Duchaine, B. (2010). Human face recognition ability is specific and highly heritable. Proceedings of the National Academy of Sciences, 107(11), 5238-5241.
- Manzoor, S. (5 November 2016). You look familiar: On patrol with the Met’s super-recognisers. The Guardian. https://www.theguardian.com/uk-news/2016/nov/05/metropolitan-police-super-recognisers
- Davis, J. P. (2019). The worldwide impact of identifying super-recognisers in police and business. The Cognitive Psychology Bulletin; Journal of the British Psychological Society: Cognitive Section, 4, 17-22.
- O’Keefe, P. (22 August 2016). The detectives who never forget a face. New Yorker. http://www.newyorker.com/magazine/2016/08/22/londons-super-recognizer-police-force
- Phillips, P. J., Yates, A. N., Hu, Y., Hahn, C. A., Noyes, E., Jackson, K., … & Chen, J. C. (2018). Face recognition accuracy of forensic examiners, superrecognizers, and face recognition algorithms. Proceedings of the National Academy of Sciences, 115(24), 6171-6176.
- [18] White, D., Kemp, R. I., Jenkins, R., Matheson, M., & Burton, A. M. (2014). Passport officers’ errors in face matching. PLOS One, 9(8), e103510.
- [19] Schofield, H. (2019). The fake French minister in a silicone mask who stole millions. Retrieved 23rd March 2020 from https://www.bbc.co.uk/news/world-europe-48510027.
- Robertson, D. J., Mungall, A., Watson, D. G., Wade, K. A., Nightingale, S. J., & Butler, S. (2018). Detecting morphed passport photos: a training and individual differences approach. Cognitive research: principles and implications, 3(1), 1-11.
- Kramer, R. S., Mireku, M. O., Flack, T. R., & Ritchie, K. L. (2019). Face morphing attacks: Investigating detection with humans and computers. Cognitive Research: Principles and Implications, 4(1), 28.
- del Rio, J. S., Moctezuma, D., Conde, C., de Diego, I. M., & Cabello, E. (2016). Automated border control e-gates and facial recognition systems. Computers & Security, 62, 49-72.
- Vine, J. (2011). Inspection of border control operations at Terminal 3, Heathrow Airport. Retrieved 23rd March 2020 from, http://icinspector.independent.gov.uk/inspections/inspection-reports/2012-inspection-reports/
- Big Brother Watch (10 February 2020). Big Brother Watch response to Met’s first operational facial recognition deployment. https://bigbrotherwatch.org.uk/2020/02/big-brother-watch-response-to-mets-first-operational-facial-recognition-deployment/
- Grother, P., Ngan, M., & Hanaoka, K. (2019). Face Recognition Vendor Test (FRVT) Part 3: Demographic Effects. National Institute of Science and Technology (NIST) NISTIR, 8280.
- Fussey, P., & Murray, D. (2019). Independent report on the London Metropolitan Police Service’s trial of live facial recognition technology. University of Essex, https://48ba3m4eh2bf2sksp43rq8kk-wpengine.netdna-ssl.com/wp-content/uploads/2019/07/London-Met-Police-Trial-of-Facial-Recognition-Tech-Report.pdf
- White, D., Burton, A. M., Kemp, R. I., Jenkins, R. (2013). Crowd effects in unfamiliar face matching. Applied Cognitive Psychology, 27(6), 769–777.
- Davis, J. P., Maigut, A., & Forrest, C. L. D. (2019). The wisdom of the crowd: A case of post- to ante-mortem face matching by police super-recognisers. Forensic Science International, 109910.
- Ramon, M., Bobak, A., & White, D. (2019). Super-recognizers: From the lab to the world and back again. British Journal of Psychology, 110(3), 461-479.