Current sensors capable of detecting chemical, biological, radiological, and nuclear agents are limited by range, volume, and environment. Due to these limitations, researchers at the Georgia Institute of Technology (Georgia Tech) are developing a biomimetic nose capable of detecting trace amounts of agents both in air and in liquids and at distances greater than currently possible. Agencies within the Department of Defense (DoD) and the Department of Homeland Security, such as Edgewood Chemical Biological Center, Defense Threat Reduction Agency, U.S. Coast Guard, and U.S. Customs and Border Protection, rely on sensors to protect people, animals, and food products from contamination and to impede drug trafficking and smuggling of banned items. In many cases, sensors are used for early detection of possible chemical/ biological agents, providing early warning of these threats. However, as good as current sensor technology has become, there are still significant limitations that can hinder security and military operations.
For example, ion and mass spectrometry separates ions based on their mobility and mass-to-charge ratio and can even detect trace amounts of fentanyl wiped off a surface [1]. Colorimetric sensor arrays change color when exposed to chemical aerosols [2-4], and microcantilever devices produce a measurable deflection due to the gas causing a chemical reaction, surface stress, or resonant frequency change [5]. For liquids, methods such as fluorescence quenching for explosive detection [6] and microfluidics for medical diagnosis [7] have shown much promise.
Despite these advances, a universal challenge remains—these sensors are proximity limited. They must be placed close to the target chemical or the chemical must be placed directly on the sensor, and detection is only possible if the chemical is present in sufficient concentrations. This is a significant limitation of modern sensors because the location and/or presence of target chemicals is often unknown, such as in the detection of narcotics at an airport or explosives in the field. Moreover, a more advanced challenge is the detection of contaminants underwater, an application that would be useful for port and harbor security, pipeline management, and securing public drinking water. However, contaminants (such as chlorine and salt) present in liquids (i.e., drinking water and seawater) cause existing underwater sensors to degrade over time, requiring the sensor to be replaced or recalibrated. With these challenges still outstanding, researchers at Georgia Tech have taken a step toward countering traditional limitations associated with sensors used for chemical detection.
Gaseous Recognition Oscillatory Machine Integrating Technology
Olfaction is defined as the chemoreception that forms the sense of smell. In 1964, the first device to perform olfaction was built using microelectrodes [8,9]. The device was only capable of registering a change between differing volatiles. It could not classify or identify the odorant, which is the contemporary goal of machine olfaction. In 1988, the term “electronic nose” was introduced at a conference in the U.K. [10], and the term has evolved to include any device intended to detect odors using a sensor array [11]. Current goals in the field of chemical detection include reducing device size, decreasing cost, enhancing sensitivity using multi-sensor arrays (such as those found in “nose-on-a-chip” technologies [12]), and improving signal processing [13]. Work by scientists at both the National Institute of Standards and Technology and the Food and Drug Administration has focused on improving the detection sensitivity of sensors by replicating the mechanism seen in canine sniffing [14]. Researchers at Georgia Tech attempted to advance the capabilities of detection even further by combining the use of this phenomenon with machine learning, developing a new type of biomimetic nose, which features enhanced sensitivity for applications in both air and underwater.
At a conference last year, the Georgia Tech team participated in a student competition to design and build an electronic nose capable of distinguishing multiple types of cheeses [15]. Competing teams were restricted to the use of the same type of simple, inexpensive, non-selective metal oxide sensor, which required significant modifications in post-processing by Georgia Tech researchers. This limitation led researchers to incorporate machine learning and a pre-concentrator into the electronic nose design. Although the goal of the competition was to develop a device capable of distinguishing between types of cheese, the underlying science remains the same regardless of the sample tested. Therefore, this technique may be capable of improving the sensitivity of detecting airborne particles, such as TNT, PETN, and RDX from explosives [16], lending itself to DoD- and DHS-relevant applications.
A review of studies regarding mammalian olfaction revealed that mammals sniff at a frequency ranging from 2 to 10 hertz (Hz) [17-20]. Therefore, researchers sought to mimic this range with Gaseous Recognition Oscillatory Machine Integrating Technology (G.R.O.M.I.T.), which can be set to sniff at a specific frequency. In order to mimic the mechanics of a sniff, Georgia Tech researchers developed a 3-D printed diaphragm pump resembling a bellows (see Figure 1), making the motion of air repeatable and controllable, evacuating the bellows by moving air at a specific velocity and frequency. Given the cross-sectional area of the tubing, At, the corresponding equation is ΔV= (U A_t)/f where ΔV is the volume change of the bellows, U is the desired air velocity, and f is the desired frequency of sniffing. For initial testing, the device was set to mimic the frequency/velocity of a dog’s sniff—a frequency of 5 Hz and velocity of 1.5 meters per second (m/s) [19] (see Figure 2). The pump can move air at a speed of up to 5.5 m/s and within the aforementioned frequency range. A section for the sample requiring detection and a previously calibrated metal oxide sensor are in series with the pump [21]. The entire system is controlled by two microcontrollers and computer program, as illustrated in Figure 3. G.R.O.M.I.T. may be the first step in identifying how sniffing improves detection. While a number of theories exist, there is no clear consensus, but using G.R.O.M.I.T. in experiments may help to test competing hypotheses. The Georgia Tech team hypothesizes that sniffing can temporarily increase the concentration of target particles.
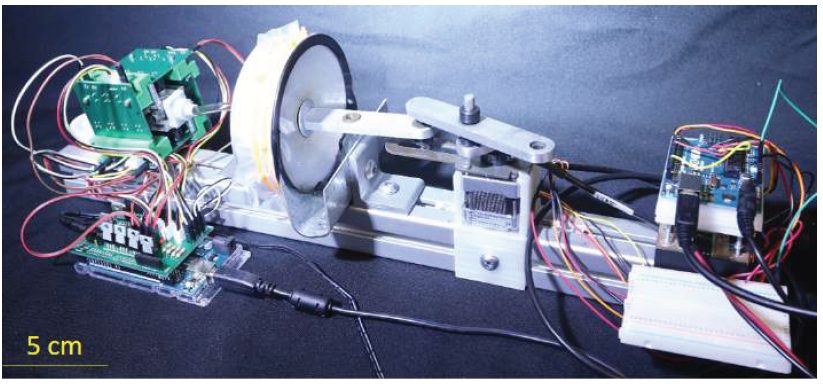
Figure 1: G.R.O.M.I.T. electronic nose
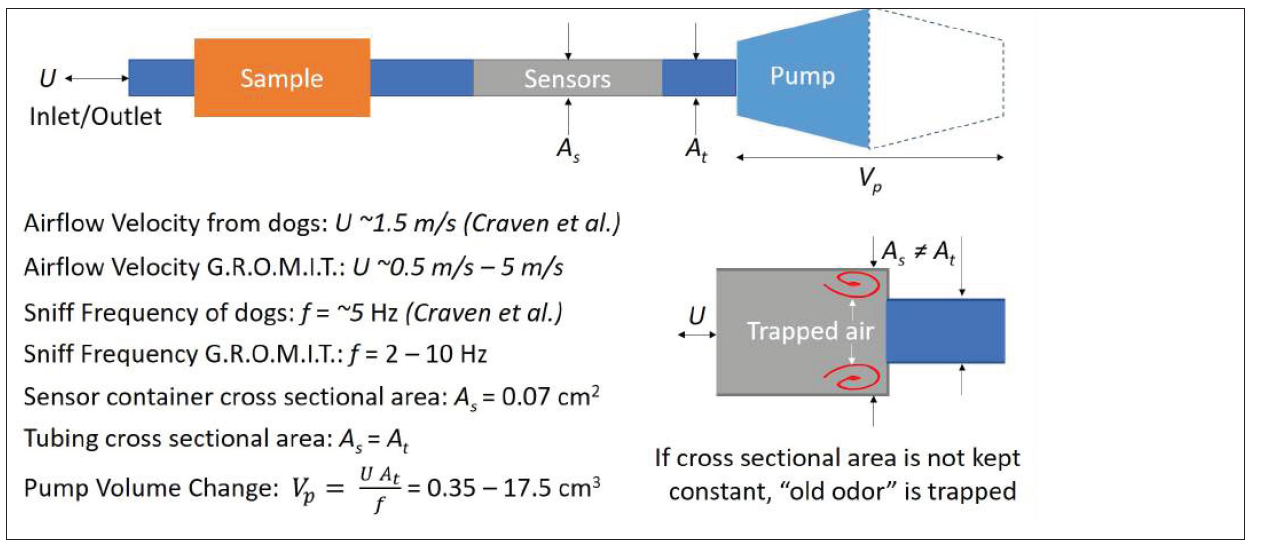
Figure 2: G.R.O.M.I.T. physical constraints
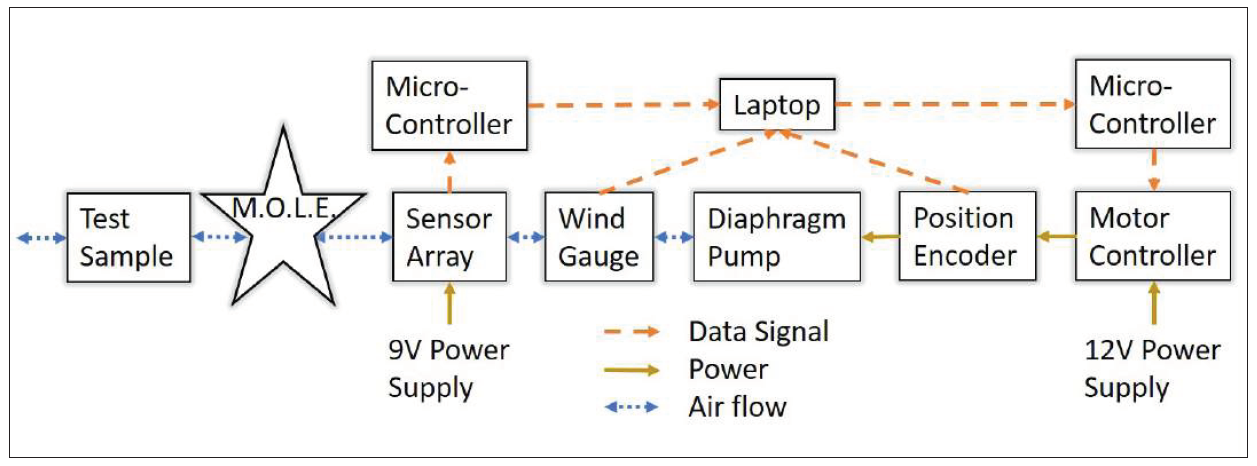
Figure 3: G.R.O.M.I.T. data, power, and air flow diagram
In a typical sensing system, air is either stagnant or moved quickly across the sensors causing a decrease in pressure [22]. Both possibilities are disadvantageous to sensing, but sniffing can avoid this problem. The transition from inhalation to exhalation in sniffing causes fluids (e.g., air and water) to reverse direction, creating a brief spike in internal pressure. This pressure brings the chemical molecules closer together, maximizing their number in the detection volume of the sensor. Moreover, larger particles respond less to these pressure fluctuations because it takes a greater force to overcome the inertia of such particles [23]. Therefore, sniffing affords the ability to perform particle size discernment whereas traditional sensing systems rely on chemical reactions alone. To take advantage of the brief period (the time between inhalation and exhalation) of high particle count, the G.R.O.M.I.T. needs a brain, which was created utilizing machine learning.
Machine learning allows computers to find their own solutions to problems without the programmer writing the solutions out. This can be done by separating complex tasks into simpler tasks that the computer can handle, such as grouping things based on a set of metrics. For example, humans categorize produce as vegetables or fruits—a delineation based on the sugar content of food. Similarly, in machine olfaction, the computer is presented the task of identifying various odors. To accomplish this task, the programs group data points based on a set of metrics. However, the central challenge of machine olfaction is in identifying these metrics, referred to as features, based on the data collected.
As G.R.O.M.I.T. “sniffs,” the odorants displace oxygen directly atop the sensor, in turn changing the sensor’s voltage. These voltage readings on their own are of little use. Instead, relevant features must be measured from them. In the produce example, the relevant feature was the sugar content of the produce. During recent testing of G.R.O.M.I.T. [15], a signature unique to each type of cheese was derived from three features: the rate at which the voltage readings change, the variation in these changes, and the rate at which the odorant was expelled from the system [20]. These features allowed G.R.O.M.I.T. to group similar odors as coming from the same source. If each feature represents an axis in three-dimensional space, then every odor sample can be assigned a position in space based on G.R.O.M.I.T.’s measurements. A Nearest Neighbors algorithm was applied to group the samples based on their distance to other samples, and then a Support Vector Machine algorithm forms boundaries which establish the training data. This training data is G.R.O.M.I.T.’s life experience—in other words, as G.R.O.M.I.T. is given a new sample, it can identify the source based on what it has previously identified. The system proved to be a successful proof of concept, successfully distinguishing 83 percent of cheese pairings [15]. Further research is needed to better distinguish between samples with nearly identical
chemical composition. The success rate could be improved by replacing the existing rudimentary sensor with a more advanced sensor, such as microcantilever sensor or colorimetric sensor array.
Underwater Detection
Machine olfaction has generally been used for air-based applications, but there are many potential applications for employing this technique underwater, including monitoring ship hulls, entrances to harbors, and within critical pipelines. However, several challenges exist with sensing in an all-liquid environment. Specifically, submergence gives rise to unwanted biofilms that impede measurements [24], and overexposure to harsh chemicals requires sensor recalibration after only a few days of use [25]. However, nature may offer solutions to overcoming these challenges. Georgia Tech researchers drew inspiration from a number of species of semi-aquatic mammals that have the ability to smell underwater: star-nosed moles (see Figure 4a), American water shrews [26], and Russian desmans [27]. These rodents track the scent of their prey at the bottom of swamps by utilizing coordinated sniffing. They blow bubbles out their noses, quickly sucking them back in before the bubble can float away. The inhaled bubble is coated with molecules of the target smell, enabling the animal to pinpoint the target’s location.
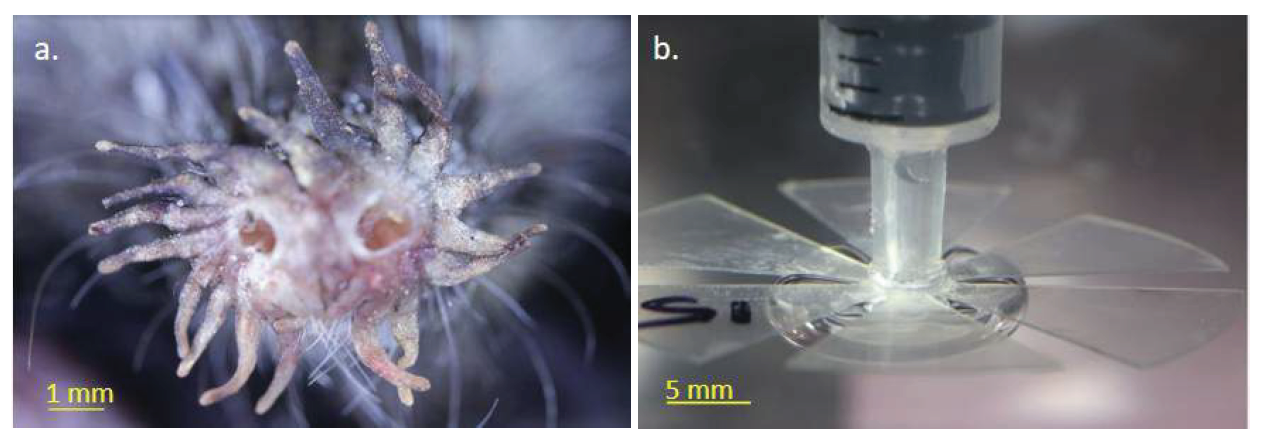
Figure 4: (A) Star nose mole and (B) M.O.L.E. attachment for use with G.R.O.M.I.T.
Based on the anatomy of the star-nose mole, Georgia Tech researchers developed an attachment, the Marine Olfaction Laser-cut End-effector (M.O.L.E.), for use with G.R.O.M.I.T that can be submerged in a liquid without encountering the aforementioned challenges. The Georgia Tech team investigated how the unique shape of the star-nosed mole allows it to perform bubble-based sniffing. Researchers developed laser-cut plastic stars mimicking a mole nose and affixed them to syringes which, like the mole, blow bubbles underwater (see Figure 4b). By testing a range of star shapes, the team elucidated how the star shape prevents bubbles from escaping—bubbles are stabilized by deformation by the arms of the star. As a mole blows a bubble, the bubble tries to rise through the gaps in the star while surface tension tries to minimize the bubble’s surface area. Arms at a proper spacing cause a bubble to stay balanced on the star, even as the mole moves around during sniffing. Researchers fitted G.R.O.M.I.T. with the plastic star shapes and found that bubbles from multiple sniffs could be stabilized. Such star-shaped adapters would allow G.R.O.M.I.T. to stabilize bubbles long enough for chemicals to diffuse into them. By mimicking the distinct shape of a mole nose, standard gas sensors can be employed rather than traditionally used sensors that must be immersed in a target liquid. The M.O.L.E. can be submerged, while still connected to G.R.O.M.I.T. stationed a distance away at a dry location. Together, the G.R.O.M.I.T. and M.O.L.E. are the first step in the design of an electronic nose capable of extended underwater detection.
Conclusion
The G.R.O.M.I.T. is a novel device that provides enhanced chemical detection and characterization. It employs machine learning algorithms to continually improve agent characterization and also mimics animal sniffing. Although current detection methods (e.g., long inhales or exposure to stagnant air) face limitations regarding sample proximity, the G.R.O.M.I.T. may fill this gap. Additionally, the M.O.L.E. allows for enhanced chemical detection underwater. Further research is required to determine if employing a higher quality sensor can enhance machine olfaction and also to evaluate alternative methods for introducing chemical samples into the sensor. The G.R.O.M.I.T. and M.O.L.E. may prove a valuable, versatile tool for CBRN threat detection in air and underwater with applications ranging from military use (such as maritime and active combat operations) to critical infrastructure protection.
References
1. Sisco, E., Verkouteren, J., Staymates, J., & Lawrence, J. (2017). Rapid detection of fentanyl, fentanyl analogues, and opioids for on-site or laboratory based drug seizure screening using thermal desorption DARTMS and ion mobility spectrometry. Forensic Chemistry, 4, 108-115. doi: 10.1016/j. forc.2017.04.001
2. Berliner, A., Lee, M.-G., Zhang, Y., Park, S. H., Martino, R., Rhodes, P. A., . . . Lim, S. H. (2014). A patterned colorimetric sensor array for rapid detection of TNT at ppt level. RSC Advances, 4(21), 10672-10675. doi:10.1039/c3ra47152g
3. Diehl, K. L., & Anslyn, E. V. (2013). Array sensing using optical methods for detection of chemical and biological hazards. Chemical Society Reviews, 42(22), 8596-8611. doi:10.1039/C3CS60136F
4. Oh, J.-W., Chung, W.-J., Heo, K., Jin, H.-E., Lee, B. Y., Wang, E., . . . Kim, C. (2014). Biomimetic virus-based colourimetric sensors. Nature Communications, 5, 3043. doi:10.1038/ncomms4043
5. Boisen, A., Dohn, S., Keller, S. S., Schmid, S., & Tenje, M. (2011). Cantilever-like micromechanical sensors. Reports on Progress in Physics, 74(3), 036101. doi:10.1088/0034-4885/74/3/036101
6. Zarei, A. R., & Ghazanchayi, B. (2016). Design and fabrication of optical chemical sensor for detection of nitroaromatic explosives based on fluorescence quenching of phenol red immobilized poly (vinyl alcohol) membrane. Talanta, 150, 162-168. doi:10.1016/j. talanta.2015.12.014
7. Dungchai, W., Chailapakul, O., & Henry, C. S. (2009). Electrochemical detection for paper-based microfluidics. Analytical Chemistry, 81(14), 5821-5826. doi:10.1021/ ac9007573
8. Gardner, J. W., Bartlett, P., Dodd, G., & Shurmer, H. (1988). Pattern recognition in the Warwick electronic nose. Paper presented at the 8th Int. Congress of the European Chemoreception Research Organisation.
9. Wilkens, W. F., & Hartman, J. D. (1964). An electronic analog for the olfactory processes. Journal of Food Science, 29(3), 372-378. doi:10.1111/j.1365-2621.1964.tb01746.x
10. Gardner, J. W., Bartlett, P., Dodd, G., & Shurmer, H. (1988). Pattern recognition in the Warwick electronic nose. Paper presented at the 8th Int. Congress of the European Chemoreception Research Organisation.
11. Pearce, T. C., Schiffman, S. S., Nagle, H. T., & Gardner, J. W. (2006). Handbook of machine olfaction: Electronic nose technology. John Wiley & Sons.
12. Datta-Chaudhuri, T., Araneda, R. C., Abshire, P., & Smela, E. (2016). Olfaction on a chip. Sensors and Actuators B: Chemical, 235, 74-78. doi:10.1016/j.snb.2016.05.048
13. Sarkar, S. T., Bhondekar, A. P., Macaš, M., Kumar, R., Kaur, R., Sharma, A., . . . Kumar, A. (2015). Towards biological plausibility of electronic noses: A spiking neural network based approach for tea odour classification. Neural Networks, 71, 142-149. doi:10.1016/j.neunet.2015.07.014
14. Staymates, M. E., MacCrehan, W. A., Staymates, J. L., Kunz, R. R., Mendum, T., Ong, T.-H., . . . Craven, B. A. (2016). Biomimetic sniffing improves the detection performance of a 3D printed nose of a dog and a commercial trace vapor detector. Scientific Reports, 6(1). doi:10.1038/srep36876
15. Spencer, T. L., Lee, A. B., & Hu, D. L. (2017, May). Sniffest 2017. Competition participation at the 2017 ISOCS/IEEE International Symposium on Olfaction and Electronic Nose (ISOEN), Montreal, Quebec, Canada.
16. Brudzewski, K., Osowski, S., & Pawlowski, W. (2012). Metal oxide sensor arrays for detection of explosives at sub-parts-per million concentration levels by the differential electronic nose. Sensors and Actuators B: Chemical, 161(1), 528-533. doi:10.1016/j. snb.2011.10.071
17. Wesson, D. W., Donahou, T. N., Johnson, M. O., & Wachowiak, M. (2008). Sniffing behavior of mice during performance in odor-guided tasks. Chemical Senses, 33(7), 581-596. doi:10.1093/chemse/bjn029
18. Xi, J., Si, X. A., Kim, J., Zhang, Y., Jacob, R. E., Kabilan, S., & Corley, R. A. (2016). Anatomical details of the rabbit nasal passages and their implications in breathing, air conditioning, and olfaction. The Anatomical Record, 299(7), 853-868. doi:10.1002/ar.23367
19. Craven, B. A., Paterson, E. G., & Settles, G. S. (2009). The fluid dynamics of canine olfaction: Unique nasal airflow patterns as an explanation of macrosmia. Journal of The Royal Society Interface, 7(47), doi:10.1098/ rsif.2009.0490
20. Lee, A. B., Spencer, T. L., Pillarisetti, J., Ersted, M., & Hu, D. L. (2017). Mimicking sniffing for improving machine olfaction. 2017 ISOCS/IEEE International Symposium on Olfaction and Electronic Nose (ISOEN). doi:10.1109/isoen.2017.7968894
21. Rodriguez-Lujan, I., Fonollosa, J., Vergara, A., Homer, M., & Huerta, R. (2014). On the calibration of sensor arrays for pattern recognition using the minimal number of experiments. Chemometrics and Intelligent Laboratory Systems, 130, 123-134. doi:10.1016/j.chemolab.2013.10.012
22. Young, D. F., Munson, B. R., Okiishi, T. H., &Huebsch, W. W. (2010). A brief introduction to fluid mechanics. John Wiley & Sons.
23. Spielman, L. A. (1977). Particle capture from low-speed laminar flows. Annual Review of Fluid Mechanics, 9(1), 297-319. doi:10.1146/annurev.fl.09.010177.001501
24. Mattila‐Sandholm, T., & Wirtanen, G. (1992). Biofilm formation in the industry: A review. Food Reviews International, 8(4), 573-603. doi:10.1080/87559129209540953
25. Hall, J., Zaffiro, A. D., Marx, R. B., Kefauver, P. C., Krishnan, E. R., Haught, R. C., & Herrmann, J. G. (2007). On-line water quality parameters as indicators of distribution system contamination. Journal of the American Water Works Association, 99(1), 66-77.
26. Catania, K. C. (2006). Olfaction: Underwater ‘sniffing’ by semi-aquatic mammals. Nature, 444(7122), 1024-1025. doi:10.1038/4441024a
27. Ivlev, Y. F., Rutovskaya, M., & Luchkina, O. (2013). The use of olfaction by the Russian desman (Desmana moschata L.) during underwater swimming. Paper presented at the Doklady Biological Sciences. References